The Importance of Bounding Boxes in Data Annotation
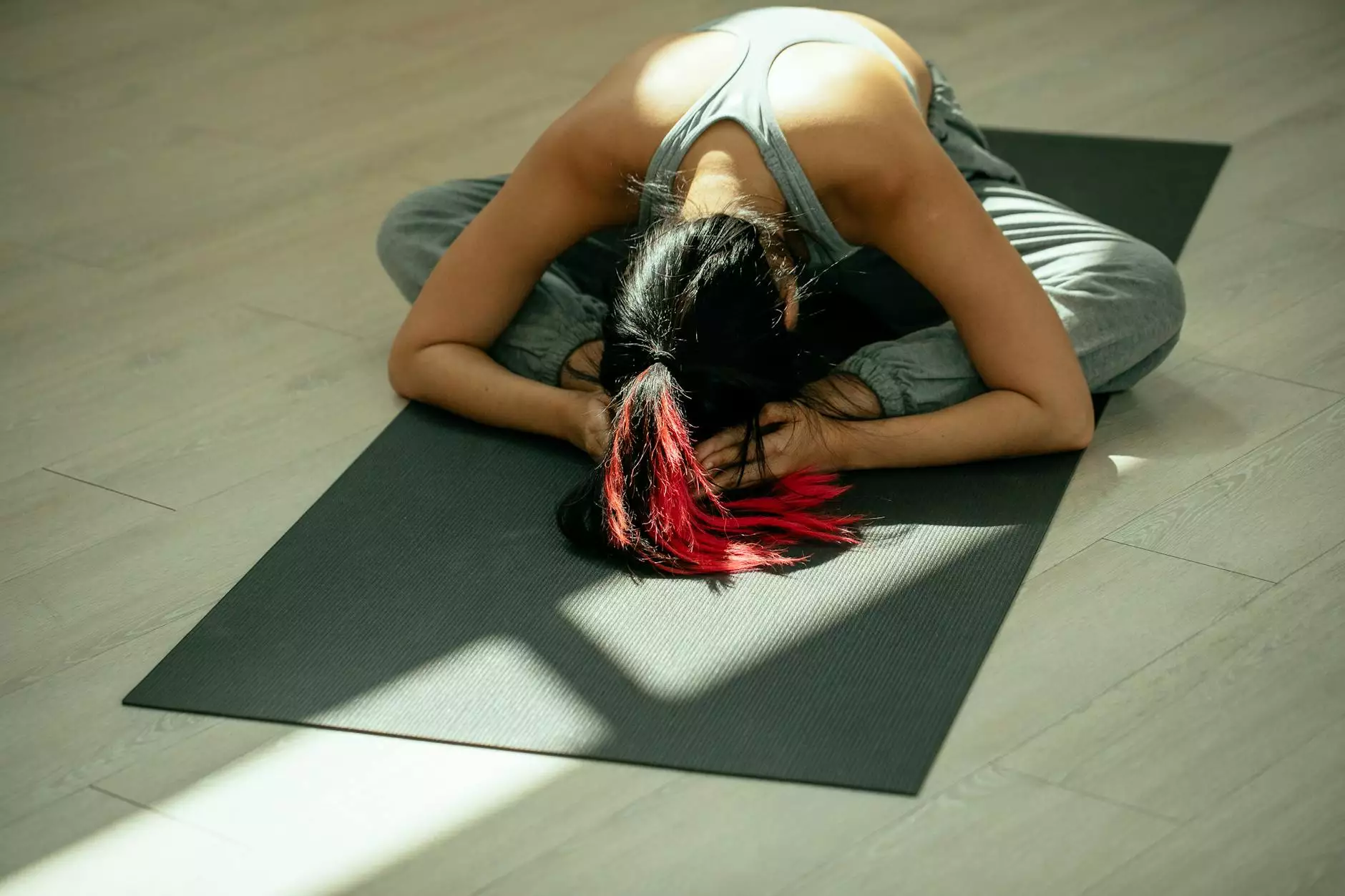
Bounding boxes are fundamental tools used in various fields, particularly in data annotation. They serve as a guiding framework that aids in the identification of important features within images and videos. This article will delve into the multi-faceted applications of bounding boxes, their significance in advanced data annotation platforms, and how they impact the burgeoning field of artificial intelligence.
Understanding Bounding Boxes
A bounding box is defined as a rectangular box that tightly encloses an object or region of interest within a digital image or video. These boxes are characterized by their x and y coordinates, which denote the box's corners. By framing objects in an image, bounding boxes simplify the process of data labeling and significantly enhance the efficiency of machine learning algorithms.
The Role of Bounding Boxes in Data Annotation Tools
Data annotation involves a systematic approach to label data accurately for machine learning models, and bounding boxes play a pivotal role. Here is why they are essential:
- Object Detection: Bounding boxes are primarily used in object detection tasks. When training a model to identify objects, annotated bounding boxes provide the necessary context and precision to the algorithm.
- Image Segmentation: They assist in image segmentation efforts, allowing models to identify the specific segments that represent distinct objects or patterns within images.
- Enhanced Accuracy: Implementing precise bounding boxes increases the accuracy of machine learning models, as they can focus on relevant areas and ignore irrelevant information.
- Scalability: As datasets grow, the scalability of data annotation becomes crucial. Bounding box methods are relatively simple and can be scaled effectively for larger datasets.
Applications of Bounding Boxes Across Industries
Bounding boxes are not limited to a single field; their versatility allows them to be applicable across a plethora of industries, including:
1. Computer Vision
In computer vision, bounding boxes are a standard practice for training algorithms that power various applications, from facial recognition systems to autonomous vehicles. Here, bounding boxes help in recognizing and localizing objects, enabling machines to interpret visual data effectively.
2. Graphic Design
Graphic designers utilize bounding boxes to help structure their layouts and compositions. By understanding where elements visually reside within a space, designers can create aesthetically pleasing and functional designs, whether for digital platforms or print media.
3. Medical Imaging
In the field of healthcare, bounding boxes are employed in analyzing medical images, such as MRIs and CT scans. These tools help radiologists identify tumors or anomalies by providing a clear delineation of areas that require closer inspection.
4. Retail and E-commerce
Bounding boxes are integral to visual search and recommendation systems in online retail. By annotating product images with bounding boxes, e-commerce platforms can enhance product visibility and improve user experience through better search results.
Benefits of Using Bounding Boxes in Machine Learning
The adoption of bounding boxes enhances machine learning processes in several compelling ways:
- Increased Efficiency: Automation and semi-automation of annotation processes through bounding boxes reduce the time spent on manual labeling.
- High-quality Datasets: By ensuring that annotations are precise, bounding boxes help in generating high-quality datasets critical for training robust models.
- Flexibility: Bounding boxes can be adjusted according to the object size and shape, allowing for flexibility in data annotation.
Implementing Bounding Boxes: A Step-by-Step Guide
Creating effective bounding boxes involves a straightforward process that can significantly impact the output quality of machine learning models. Here is a step-by-step guide:
Step 1: Select the Right Tool
Choose a data annotation tool that supports bounding box annotations. Tools like Keylabs.ai provide efficient platforms that streamline the annotation process.
Step 2: Upload the Dataset
Upload the images or videos requiring annotation to the platform. An organized dataset is essential for accurate and effective bounding box placement.
Step 3: Define the Bounding Boxes
Manually or semi-automatically draw bounding boxes around the objects of interest. Ensure that each box is tight and only encompasses the object it is meant to represent.
Step 4: Review and Validate
Once the bounding boxes are defined, conduct a thorough review to validate accuracy. Conduct quality checks to ensure they reflect the correct objects accurately.
Step 5: Export Annotations
After validation, export the annotations in the required format for use in training machine learning models. Properly formatted output is crucial for seamless integration into AI systems.
The Future of Bounding Boxes in AI
As artificial intelligence continues to evolve, the role of bounding boxes remains pivotal. The development of more advanced algorithms necessitates increasingly precise data annotation methods. In tandem with machine learning advancements, we can expect innovations in bounding box generation and annotation processes, propelling industries forward.
Innovative Technologies
Emerging technologies such as automated data annotation and deep learning techniques will likely reshape how bounding boxes are deployed in the future. Automated systems specifically designed for generating bounding boxes have the potential to drastically reduce the manual effort required while maintaining or improving accuracy levels.
Conclusion
Bounding boxes are more than mere rectangles; they are a crucial element of the modern data annotation landscape. As industries increasingly rely on precise data labels to train AI models, understanding and implementing bounding boxes will remain a valuable skill. By leveraging effective data annotation tools like Keylabs.ai, businesses can enhance their efficiency and accuracy, ultimately positioning themselves at the forefront of AI advancements.
As we look to the future, bounding boxes will play an essential role in determining how well machines can interpret visual data, thus influencing the trajectory of technological innovation in numerous fields.